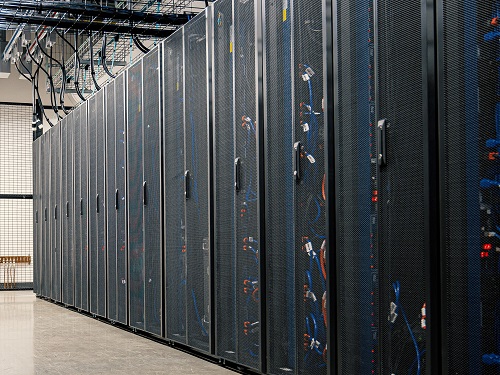
Generative AI tools like ChatGPT plus the rapid and revolutionary growth of AI are changing the face of most industries and generating dire warnings about the future, but what about the effects on datacentres?
Data Centres And Their Importance
Data centres are the specialised facilities that house a large number of computer servers and networking equipment, serving as centralised locations where businesses and organisations store, manage, process, and distribute their digital data. These facilities are designed to provide a secure, controlled environment for storing and managing vast amounts of data in the cloud.
In our digital, cloud computing business world, data centres, therefore play a crucial role in supporting various industries and services that rely on large-scale data processing and storage, and are utilised by organisations ranging from small businesses to multinational corporations, cloud service providers, internet companies, government agencies, and research institutions.
The Impacts of Generative AI
There are number of ways that generative AI is impacting data centres. These include:
– The need for more data centres. Generative AI applications require significant computational resources, including servers, GPUs, and data storage devices. As the adoption of generative AI grows, data centres will need to invest and expand their infrastructure to accommodate the increased demand for processing power and storage capacity and this will change the data centre landscape. For example, greater investment in (and greater numbers of) data centres will be needed. It’s been noted that the massive data-crunching requirements of AI platforms like ChatGPT, for example, couldn’t continue to operate without using Microsoft’s (soon-to-be-updated) Azure cloud platform. This has led to Microsoft now building a new 750K SF hyperscale data centre campus near Quincy, WA, to house three 250K SF server farms on land costing $9.2M. Presumably, with more data centres there will also need to be greater efforts and investment to reduce and offset their carbon consumption.
– Greater power consumption and more cooling needed. Generative AI models are computationally intensive and consume substantial amounts of power. Data centres also have backup power sources to ensure a smooth supply, such as uninterruptible power supplies (UPS) and generators. With more use of generative AI, data centres will need to ensure they have sufficient power supply and cooling infrastructure to support the energy demands of generative AI applications. This could mean that data centres will now need to improve power supplies to cope with the demands of generative AI by conducting power capacity planning, upgrading infrastructure, implementing redundancy and backup systems, optimising power distribution efficiency, integrating renewable energy sources, implementing power monitoring and management systems, and collaborating with power suppliers. These measures could enhance power capacity, reliability, efficiency, and sustainability. More data centres may also need to be built with their own power plants (like Microsoft did in Dublin in 2017).
In terms of the greater need for cooling, i.e. to improve cooling capacity for generative AI in data centres, strategies include optimising airflow management, adopting advanced cooling technologies like liquid cooling, implementing intelligent monitoring systems, utilising computational fluid dynamics simulations, exploring innovative architectural designs, and leveraging AI algorithms for cooling control optimisation. These measures could all enhance airflow efficiency, prevent hotspots, improve heat dissipation, proactively adjust cooling parameters, inform cooling infrastructure design, and dynamically adapt to workload demands to meet the cooling challenges posed by generative AI.
– The need for scalability and flexibility. Generative AI models often require distributed computing and parallel processing to handle the complexity of training and inference tasks. Data centres therefore need to provide scalable and flexible infrastructure that can efficiently handle the workload and accommodate the growth of generative AI applications. Data centres will, therefore, need to be able to support generative AI applications through means such as:
– Virtualisation for dynamic resource allocation.
– High-performance Computing (HPC) clusters for computational power.
– Distributed storage systems for large datasets.
– Enhanced network infrastructure for increased data transfer.
– Edge computing for reduced latency and real-time processing.
– Containerisation platforms for flexible deployment and resource management.
– Data storage and retrieval. Generative AI models require extensive amounts of training data, which must be stored and accessed efficiently. Data centres now need to be able to optimise their data storage and retrieval systems to handle large datasets and enable high-throughput training of AI models.
– Security and privacy. Generative AI introduces new security and privacy challenges. Data centres must now be able to ensure the protection of sensitive data used in training and inferencing processes. Additionally, they need to address potential vulnerabilities associated with generative AI, such as the generation of realistic but malicious content or the potential for data leakage. Generative AI also poses cybersecurity challenges, as it can be used to create vast quantities of believable phishing emails or generate code with security vulnerabilities. Rather than just relying upon a lot of verification, there is likely to be increased dependency on skilled workers and smart software may be necessary to address these security risks effectively.
– Customisation and integration. Generative AI models often require customisation and integration into existing workflows and applications. This means that data centres need to provide the necessary tools and support for organisations to effectively integrate generative AI into their systems and leverage its capabilities.
– Skillset requirements. Managing and maintaining generative AI infrastructure requires specialised skills and data centres will need to invest in training their personnel and/or attracting professionals with expertise in AI technologies to effectively operate and optimise the infrastructure supporting generative AI.
– Optimisation for AI workloads. The rise of generative AI also means that data centres need to find ways to optimise their operations and infrastructure to cater to the specific requirements of AI workloads. This includes considerations for power efficiency, cooling systems, network bandwidth, and storage architectures that are tailored to the demands of generative AI applications.
– Uncertain infrastructure requirements. The power consumption and hardware requirements of the increasing use of generative AI applications are yet to be fully understood and this means that the impact on software and hardware remains uncertain, and the scale of infrastructure needed to support generative AI is still not clear. The implications of this for data centres are, for example:
– A lack of clarity on power consumption and hardware needs/the specific power and hardware requirements of generative AI applications are not fully understood, makes it challenging for data centres to accurately plan and allocate resources.
– The impact of generative AI on software and hardware is still unclear which makes it difficult for data centres to determine the necessary upgrades or modifications to support these applications.
– Without a clear understanding of the demands of generative AI, data centres cannot accurately estimate the scale of infrastructure required, potentially leading to under-provisioning or over-provisioning of resources.
– The need for flexibility and adaptability. Data centres must now be prepared to adjust their infrastructure dynamically to accommodate the evolving requirements of generative AI applications as more information becomes available.
AI Helping AI
Ironically, data centres could use AI itself to help optimise their operations and infrastructure. For example, through:
– Predictive maintenance. AI analysing sensor data to detect equipment failures, minimising downtime.
– Energy efficiency. AI optimising power usage, cooling, and workload placement, reducing energy waste.
– Workload Optimisation. AI maximising performance by analysing workload patterns and allocating resources efficiently.
– Anomaly Detection. AI monitoring system metrics, identifies abnormal patterns and flags security or performance issues.
– Capacity Planning. AI analysing data to predict resource demands, optimising infrastructure expansion.
– Dynamic Resource Allocation. AI dynamically scaling computing resources, storage, and network capacity based on workload demands.
What Does This Mean For Your Business?
Overall, while generative AI offers opportunities for increased efficiency and productivity for businesses, it also poses several challenges related to infrastructure, trust, security, and compliance. Data centres in our digital society and cloud-based business world now play a crucial role in supporting industries, business, services and as such, whole economies so how data centres to adapt quickly and effectively to the challenges posed by AI (or not) is something that could potentially affect all businesses going forward.
As a data centre operator or a business relying on data centres for smooth operations, the impact of generative AI on data centres presents both opportunities and challenges. On the one hand, the increased demand for processing power and storage capacity necessitates investments in infrastructure expansion and upgrades, providing potential business opportunities for data centre operators. It may lead to the establishment of more data centres and the need for greater efforts to reduce their carbon footprint.
However, this growth in generative AI also brings challenges that need to be addressed. Data centres must ensure sufficient power supply and cooling infrastructure to support the energy demands and heat dissipation requirements of generative AI applications. This may require capacity planning, infrastructure upgrades, integration of renewable energy sources, and the adoption of advanced cooling technologies. It also presents huge challenges in terms of trying to provide the necessary capacity in a way that minimises carbon emissions and meeting environmental targets.
Additionally, with the rise of generative AI, data centres now need to consider scalability, flexibility, security, and privacy implications. They must provide the necessary infrastructure and tools for businesses to integrate generative AI into their workflows and applications securely. Skillset requirements also come into play, as personnel need to be trained in AI technologies to effectively operate and optimise the data centre infrastructure.
Overall, understanding and addressing the implications of generative AI on data centres is crucial for both data centre operators and businesses relying on these facilities. By adapting to the evolving demands of generative AI and investing in optimised infrastructure and pursuing innovation, data centre operators can provide reliable and efficient services to businesses, ensuring seamless operations and unlocking the potential of generative AI for various industries.